GlobalSIP 2013 Symposium on:
Graph Signal Processing
[Download the PDF Call for Papers]
Applications ranging from the emerging smart grid to social networks, from neuroscience to transportation and telecommunication network monitoring, are giving rise to massive data sets where the data is naturally supported on a graph. In these scenarios the data associated to nodes in the graph can be viewed as a “graph signal” and it is natural to ask whether signal processing techniques and methodologies could be used for dealing with these graph signals. Graph signal processing focuses on the interplay between the graph topology and characteristics of the corresponding signals. The aim of this symposium is to bring together researchers working in the emerging field of graph signal processing to exchange ideas and present their latest results. The symposium will also serve as a forum for researchers in the signal processing community interested in learning about the latest developments in this field.Submissions of at most 4 pages in two-column IEEE format are welcome on topics including:
- Transforms for graph signals
- Estimation, denoising, and compression for graph signals
- Sparse representations of graph signals
- Multi-scale analysis on graphs
- Graph signal downsampling and simplification
- Uncertainty principles for graph signals
- Estimating graph structure from data point-clouds
- Graph signal processing in machine learning
- Applications of graph signal processing
Keynote Speakers
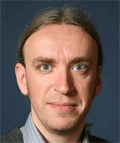
Pierre Vandergheynst, École Polytechnique Fédérale de Lausanne, Switzerland, Towards Multi-scale Signal Processing on Graphs
It is no surprise that the recent surge in applications involving large amount of network data is a challenge for signal processing and an invitation to re-invent traditional methods. In this talk I will review recent constructions of wavelets and other multi-scale transforms for data defined at the vertices of, potentially large, graphs. I will highlight interesting similarities, but also differences, with traditional digital signal processing methods such as wavelets and discuss efficient algorithms and fundamental limits. I will also showcase applications such as inference on graphs and sub-network identification using these new methods.
Pierre Vandergheynst received the M.S. degree in physics and the Ph.D. degree in mathematical physics from the Université catholique de Louvain, Louvain-la-Neuve, Belgium, in 1995 and 1998, respectively. From 1998 to 2001, he was a Postdoctoral Researcher with the Signal Processing Laboratory, Swiss Federal Institute of Technology (EPFL), Lausanne,Switzerland. He was Assistant Professor at EPFL (2002-2007), where he is now an Associate Professor.
His research focuses on harmonic analysis, sparse approximations and mathematical data processing in general with applications covering signal, image and high dimensional data processing, sensor networks, computer vision.
He was co-Editor-in-Chief of Signal Processing (2002-2006) and Associate Editor of the IEEE Transactions on Signal Processing (2007-2011), the flagship journal of the signal processing community. He has been on the Technical Committee of various conferences, serves on the steering committee of the SPARS workshop and was co-General Chairman of the EUSIPCO 2008 conference.
Pierre Vandergheynst is the author or co-author of more than 70 journal papers, one monograph and several book chapters. He has received two IEEE best paper awards.
Professor Vandergheynst is a laureate of the Apple 2007 ARTS award and of the 2009-2010 De Boelpaepe prize of the Royal Academy of Sciences of Belgium.
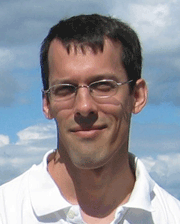
Eric Kolaczyk, Boston University, USA, Inference of Network Summary Statistics Through Network Denoising
Consider observing an undirected network that is ‘noisy’ in the sense that there are Type I and Type II errors in the observation of edges. Such errors can arise, for example, in the context of inferring gene regulatory networks in genomics or functional connectivity networks in neuroscience. Given a single observed network then, to what extent are summary statistics for that network representative of their analogues for the true underlying network?
Can we infer such statistics more accurately by taking into account the noise in the observed network edges? In this talk I will describe work in which we answer both of these questions. In particular, we develop a spectral-based methodology using the adjacency matrix to ‘denoise’ the observed network data and produce more accurate inference of the summary statistics of the true network. We characterize performance of our methodology through bounds on appropriate notions of risk in the L2 sense, and conclude by illustrating the practical impact of this work on synthetic and real-world data.
Eric Kolaczyk is Professor of Statistics, and Director of the Program in Statistics, in the Department of Mathematics and Statistics at Boston University, where he also is an affiliated faculty member in the Program in Bioinformatics, the Program in Computational Neuroscience, and the Division of Systems Engineering. Prof. Kolaczyk's main research interests currently revolve around the statistical analysis of network-indexed data, and include both the development of basic methodology and inter-disciplinary work with collaborators in bioinformatics, computer science, geography, neuroscience, and sociology. Besides various research articles on these topics, he has also authored a book in this area — Statistical Analysis of Network Data: Methods and Models (Springer, 2009). He has given various short courses on material from his book in recent years, including for the Center for Disease Control (CDC) and the Statistical and Applied Mathematical Sciences Institute (SAMSI) in the US as well as similar venues in Belgium, England, and France. Prior to his working in the area of networks, Prof. Kolaczyk spent a decade working on statistical multi-scale modeling. Prof. Kolaczyk has served as associate editor on several journals, including currently the Journal of the American Statistical Association and previously the IEEE Transactions in Image Processing. He has also served as co-organizer for workshops focused on networks and network data. He is an elected fellow of the American Statistical Association (ASA), an elected senior member of the Institute for Electrical and Electronics Engineers (IEEE), and an elected member of the International Statistical Institute (ISI).
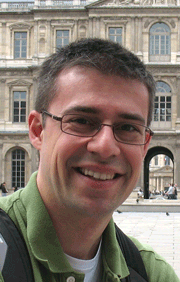
Mauro Maggioni, Duke University, USA, Multiscale Analysis of Time-Varying Graphs
Time series of graphs arise in many branches of sciences and applications. We discuss novel techniques for measuring distances between graphs, based on multiscale analysis of random walks, in a way that is both sensitive to localized changes and robust to "noisy" perturbations of the graph. From this we derive a framework for analyzing time series of graphs, together with fast algorithms. Finally, we discuss applications to families of graphs with multiscale structure, as well as real data mapped to dynamic graphs.
Mauro Maggioni works at the intersection between harmonic analysis, probability, machine learning, spectral graph theory, and signal processing. He received his B.Sc. in Mathematics summa cum laude at the Universitá degli Studi in Milan in 1999, the Ph.D. in Mathematics from the Washington University, St. Louis, in 2002. He then was a Gibbs Assistant Professor in Mathematics at Yale University till 2006, when he moved to Duke University, where is now Professor in Mathematics, Electrical and Computer Engineering, and Computer Science. He received the Popov Prize in Approximation Theory in 2007, a N.S.F. CAREER award and Sloan Fellowship in 2008, and was nominated Fellow of the American Mathematical Society in 2013. He is a member of the A.M.S. and S.I.A.M.
Paper Submission
Submit papers of at most 4 pages in two-column IEEE format through the GlobalSIP website at http://www.ieeeglobalsip.org/Papers.asp. All papers (contributed and invited) will be presented as posters.
Important Dates
Paper Submission Deadline | June 15, 2013 |
Review Results Announce | July 30, 2013 |
Camera-Ready Papers Due | September 7, 2013 |