GlobalSIP 2013 Symposium on:
Controlled Sensing For Inference: Applications, Theory and Algorithms
[Download the PDF Call for Papers]
The GlobalSIP symposium on Controlled Sensing for Inference, to be held in Austin Texas in December 2013, invites research papers from scientists, engineers, and research Scholars. The IEEE Global Conference on Signal and Information Processing (GlobalSIP), which hosts co-located symposia, is a new flagship IEEE Signal Processing Society conference that will focus on signal and information processing and up-and-coming signal processing themes.The symposium welcomes papers across several disciplines in information sciences around the common theme of Controlled Sensing for Inference, which is concerned with the control and management of different sensing assets in information gathering systems towards achieving a particular inference task. The task of developing a new science for controlled sensing is a daunting task and is only possible through the contributions and the innovations across the different disciplines of information sciences, including signal processing, information theory, network computation, control, detection and estimation and algorithms, and through the complementary roles of basic and applied research. The goal of this symposium is to bring together key researchers from different research communities to focus on the theme of controlled sensing and sensor management for inference. This symposium is a one-day symposium that will consist of 3-mini plenary talks given by reputed researchers in the field, and 2 sessions for both contributed and invited technical papers spanning a broad range of topics in basic and applied research. All technical presentations will be in the poster format. Each poster session will be 1.5-2 hours in duration, and will consist of high quality invited and contributed papers. Accepted papers will appear in the proceedings of GlobalSIP-Symposium on Controlled Sensing for Inference: Applications, Theory and Algorithms.
Submissions of at most 4 pages in two-column IEEE format are welcome on topics including:
- Sensor Management for tracking
- Sensor Management for detection, estimation, and classification
- Management of heterogeneous sensing resources
- Data-driven and non-parametric inference methods
- Information collection, processing and fusion
- Fundamental limits of sensing systems
- Applications of controlled sensing to infrastructure monitoring
- Controlled sensing for medical imaging
- Radar and surveillance applications
- Controlled sensing in social networks
Keynote Speakers
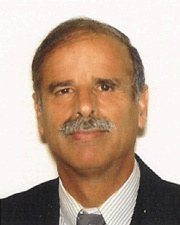
David Castañón, Boston University, Scalable Controlled Sensing for Markov Tasks
David Castañón is the Chair of the Electrical and Computer Engineering Department at Boston University. He received his Ph.D. in Applied Mathematics at Massachusetts Institute of Technology, and his B.S. in Electrical Engineering at Tulane University. Before joining Boston University, he was Chief Scientist of ALPHATECH, Inc. He has served in various executive positions for the IEEE Control Systems Society, including member of the Society's Board of Governors, Vice president for Finance, and President. He served as General Chair and Program Chair for the IEEE Control Systems Society's flagship conference, the IEEE Conference on Decision and Control, and received the Society's Distinguished Member Award in 2006. Prof. Castañón is a former member of the Air Force Scientific Advisory Board, and served as Deputy Director of the NSF Engineering Research Center for Subsurface Sensing and Imaging. He is the currently the co-director of the Center for Information and Systems Engineering at Boston University, and Associate Director of the ALERT Center of Excellence in Explosives Detection. His research interests include optimization, inverse problems, stochastic control and machine learning, with diverse applications such as target recognition, compressive sensing and tomographic image reconstruction.
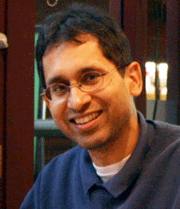
Vikram Krishnamurthy, University of British Columbia, Controlled Sensing and Social Learning
This talk describes social learning in the context of controlled sensing. Individual agents perform social learning to estimate an underlying state of nature and thereby make local decisions. How can a global decision maker use these local decisions to optimize a utility function? Two examples are considered: The first example deals with the quickest detection/estimation problem when individual agents perform social learning. The second example deals with a global decision maker that optimizes a social utility function to delay herding amongst agents. In both examples, the optimal strategy of the global decision maker is unusual in that the the stopping set is non convex. In the context of controlled sensing, these results show that global decision making based on local decisions of sensors (rather than Bayesian posteriors) can result in unusual behaviour.
Vikram Krishnamurthy received his Ph.D from the Australian National University in 1992. He is a professor and Canada Research Chair at the Department of Electrical Engineering, University of British Columbia, Vancouver, Canada. His current research interests include computational game theory, stochastic control and applications in the dynamics of protein molecules in biophysical systems. He has served as Distinguished lecturer for the IEEE signal processing society and Editor in Chief of IEEE Journal Selected Topics in Signal Processing.
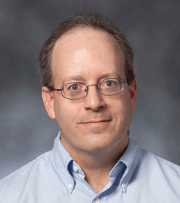
Lawrence Carin, Duke University, Adaptive Compressive Sensing for Poisson Data
Compressive sensing (CS) exploits redundancies in data to reduce the number of measurements that need be performed, relative to classic Nyquist sampling theory. Most previous CS research has assumed that the measurement noise is Gaussian, and that the projection measurements are defined at random. In this talk we reconsider both of these assumptions. First, in many sensing settings the data are Poisson, such as low-photon-count sensors, and the assumption of Gaussian noise is inappropriate. Secondly, by exploiting prior information about the statistics of the underlying data, one can use information theory to design optimal compressive measurements. In this talk we examine these issues, and demonstrate the theory on a real low-photon-count CS sensing system.
Lawrence Carin earned the BS, MS, and PhD degrees in electrical engineering at the University of Maryland, College Park, in 1985, 1986, and 1989, respectively. In 1989 he joined the Electrical Engineering Department at Polytechnic University (Brooklyn) as an Assistant Professor, and became an Associate Professor there in 1994. In September 1995 he joined the Electrical Engineering Department at Duke University, where he is now the William H. Younger Professor of Engineering. He is also now the Chairman of Electrical & Computer Engineering at Duke. He is a co-founder of Signal Innovations Group, Inc. (SIG), a small business, where he serves as the Director of Technology. His current research interests include applied statistics, information theory and machine learning. He has published over 250 peer-reviewed papers, he is an IEEE Fellow, and he is a member of the Tau Beta Pi and Eta Kappa Nu honor societies.
Paper Submission
Submit papers of at most 4 pages in two-column IEEE format through the GlobalSIP website at http://www.ieeeglobalsip.org/Papers.asp. All papers (contributed and invited) will be presented as posters.
Important Dates
Paper Submission Deadline | June 15, 2013 |
Review Results Announce | July 30, 2013 |
Camera-Ready Papers Due | September 7, 2013 |