GlobalSIP 2013 Symposium on:
New Sensing and Statistical Inference Methods
[Download the PDF Call for Papers]
We are living in interesting times. Our societies have begun to embrace the transition to a digital world in which computation and data will fuel economic developments, social changes, scientific discoveries, and technological innovations. Indeed, businesses, governments, scientists, engineers, medical professionals, etc., are increasingly relying on computation- and data-enabled methods for achieving their goals. Still, a complete transition to a world in which computation and data facilitate decision making for social and economic uplifting, and improve overall life quality requires meeting huge challenges. The Symposium on New Sensing and Statistical Inference Methods is meant to explore solutions to two of these challenges, namely, sensing and statistical inference. Both these challenges are rather grand in nature and successfully addressing them requires a concerted effort by practitioners and theoreticians working at the intersection of signal processing, statistics, harmonic analysis, machine learning and systems engineering. The motivation for this symposium arises from the need to provide a common platform for the exchange of ideas among a diverse group of researchers, with a common focus on sensing and statistical inference problems of the future.Submissions of at most 4 pages in two-column IEEE format are welcome on topics including:
- Active learning and adaptive sampling
- Compressive-sensing-inspired systems
- Computational imaging systems
- Computational methods for "big data"
- Data-adaptive representation theory/Dictionary learning
- Distributed statistics/machine learning
- High-dimensional statistical inference
- Manifold-based signal processing
- New sensing paradigms in medical imaging
- Information processing in social networks
- Robust statistical inference
- Sensing/inference for biological processes
- Sensing/processing of hyperspectral data
- Statistical inference in graphical models
Keynote Speakers
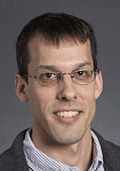
Eric Kolaczyk, Boston University, A Compressed PCA Subspace Method for Anomaly Detection in High-Dimensional Data
Random projection is widely used as a method of dimension reduction. In recent years, its combination with standard techniques of regression and classification has been explored. In this talk we present a method for its use in anomaly detection in high-dimensional settings, in conjunction with principal component analysis (PCA) and corresponding subspace detection methods. We assume a so-called spiked covariance model for the underlying data generation process and a Gaussian random projection. We adopt a hypothesis testing perspective of the anomaly detection problem, with the test statistic defined to be the magnitude of the residuals of a PCA analysis. Under the null hypothesis of no anomaly, we characterize the relative accuracy with which the mean and variance of the test statistic from compressed data approximate those of the corresponding test statistic from uncompressed data. Furthermore, under a suitable alternative hypothesis, we provide expressions that allow for a comparison of statistical power for detection. Finally, whereas these results correspond to the ideal setting in which the data covariance is known, we show that it is possible to obtain the same order of accuracy when the covariance of the compressed measurements is estimated using a sample covariance, as long as the number of measurements is of the same order of magnitude as the reduced dimensionality. We illustrate the practical impact of our results in the context of predicting volume anomalies in Internet traffic data.
Eric Kolaczyk is Professor of Statistics, and Director of the Program in Statistics, in the Department of Mathematics and Statistics at Boston University, where he also is an affiliated faculty member in the Program in Bioinformatics, the Program in Computational Neuroscience, and the Division of Systems Engineering. Prof. Kolaczyk's main research interests currently revolve around the statistical analysis of network-indexed data, and include both the development of basic methodology and inter-disciplinary work with collaborators in bioinformatics, computer science, geography, neuroscience, and sociology. Besides various research articles on these topics, he has also authored a book in this area — Statistical Analysis of Network Data: Methods and Models (Springer, 2009). He has given various short courses on material from his book in recent years, including for the Center for Disease Control (CDC) and the Statistical and Applied Mathematical Sciences Institute (SAMSI) in the US as well as similar venues in Belgium, England, and France. Prior to his working in the area of networks, Prof. Kolaczyk spent a decade working on statistical multi-scale modeling. Prof. Kolaczyk has served as associate editor on several journals, including currently the Journal of the American Statistical Association and previously the IEEE Transactions in Image Processing. He has also served as co-organizer for workshops focused on networks and network data. He is an elected fellow of the American Statistical Association (ASA), an elected senior member of the Institute for Electrical and Electronics Engineers (IEEE), and an elected member of the International Statistical Institute (ISI).
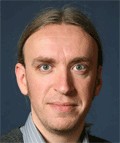
Pierre Vandergheynst, EPFL, Compressive Source Separation: Algorithms and Applications
We propose and analyze a new model for Hyperspectral Images (HSI) based on the assumption that the whole signal is composed of a linear combination of few sources, each of which has a specific spectral signature, and that the spatial abundance maps of these sources are themselves piecewise smooth and therefore efficiently encoded via typical sparse models. We derive new sampling schemes exploiting this assumption and give theoretical lower bounds on the number of measurements required to reconstruct HSI data and recover their source model parameters. This allows us to segment hyperspectral images into their source abundance maps directly from compressed measurements. We also propose efficient optimization algorithms and perform extensive experimentation on synthetic and real datasets, which reveals that our approach can be used to encode HSI with far less measurements and computational effort than traditional CS methods.
Pierre Vandergheynst received the M.S. degree in physics and the Ph.D. degree in mathematical physics from the Université catholique de Louvain, Louvain-la-Neuve, Belgium, in 1995 and 1998, respectively. From 1998 to 2001, he was a Postdoctoral Researcher with the Signal Processing Laboratory, Swiss Federal Institute of Technology (EPFL), Lausanne, Switzerland. He was Assistant Professor at EPFL (2002-2007), where he is now an Associate Professor. His research focuses on harmonic analysis, sparse approximations and mathematical data processing in general with applications covering signal, image and high dimensional data processing, sensor networks, computer vision. He was co-Editor-in-Chief of Signal Processing (2002-2006) and Associate Editor of the IEEE Transactions on Signal Processing (2007-2011), the flagship journal of the signal processing community. He has been on the Technical Committee of various conferences, serves on the steering committee of the SPARS workshop and was co-General Chairman of the EUSIPCO 2008 conference. Pierre Vandergheynst is the author or coauthor of more than 70 journal papers, one monograph and several book chapters. He has received two IEEE best paper awards. Professor Vandergheynst is a laureate of the Apple 2007 ARTS award and of the 2009-2010 De Boelpaepe prize of the Royal Academy of Sciences of Belgium.
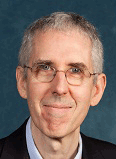
Alfred O. Hero III, University of Michigan, Spatio-temporal Graphical Models for High Dimensional Data
Graphical models provide a sparse parsimonious description of the dependency structure of multivariate data. When the data has dependency over both time and space there can be additional structure that can be exploited to reduce the complexity of the model. We will present recent approaches to exploiting such structure for Gaussian graphical models.
Alfred O. Hero III received the B.S. (summa cum laude) from Boston University (1980) and the Ph.D from Princeton University (1984), both in Electrical Engineering. Since 1984 he has been with the University of Michigan, Ann Arbor, where he is the R. Jamison and Betty Williams Professor of Engineering. His primary appointment is in the Department of Electrical Engineering and Computer Science and he also has appointments, by courtesy, in the Department of Biomedical Engineering and the Department of Statistics. In 2008 he was awarded the Digiteo Chaire d'Excellence, sponsored by Digiteo Research Park in Paris, located at the Ecole Superieure d'Electricite, Gif-sur-Yvette, France. He is a Fellow of the Institute of Electrical and Electronics Engineers (IEEE) and several of his research articles have received best paper awards. Alfred Hero was awarded the University of Michigan Distinguished Faculty Achievement Award (2011). He received the IEEE Signal Processing Society Meritorious Service Award (1998) and the IEEE Third Millenium Medal (2000). Alfred Hero was President of the IEEE Signal Processing Society (2006-2008) and was on the Board of Directors of the IEEE (2009-2011) where he served as Director of Division IX (Signals and Applications). Alfred Hero's recent research interests are in statistical signal processing, machine learning and the analysis of high dimensional spatio-temporal data. Of particular interest are applications to networks, including social networks, multi-modal sensing and tracking, database indexing and retrieval, imaging, and genomic signal processing.
Paper Submission
Submit papers of at most 4 pages in two-column IEEE format through the GlobalSIP website at http://www.ieeeglobalsip.org/Papers.asp. All papers (contributed and invited) will be presented as posters.
Important Dates
Paper Submission Deadline | June 15, 2013 |
Review Results Announce | July 30, 2013 |
Camera-Ready Papers Due | September 7, 2013 |